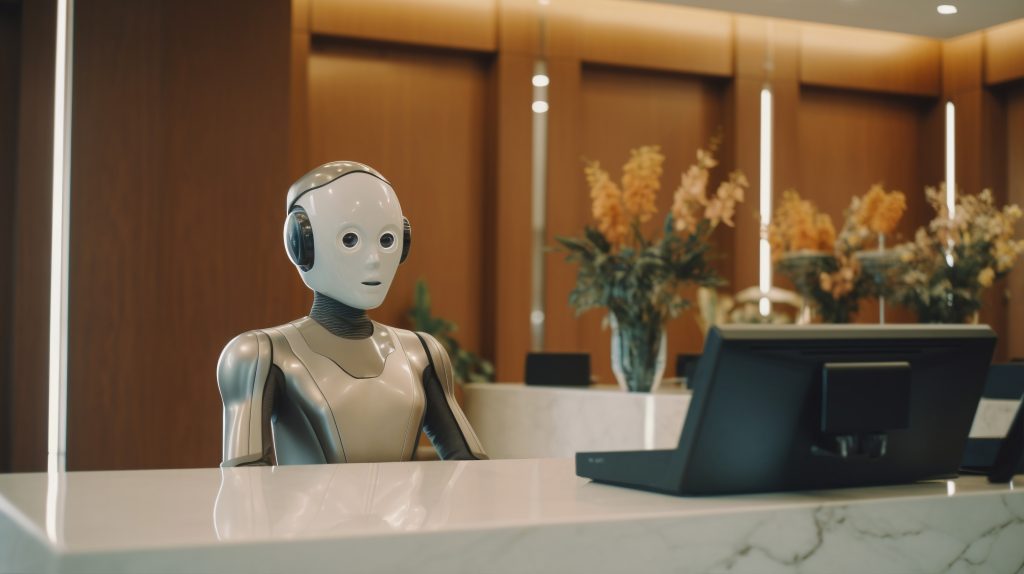
The world of artificial intelligence (AI) evolves at a dizzying pace, often leaving people overwhelmed by jargon and confused by the rapid advancements. Terms like AI agents, large language models (LLMs), and related technologies can blur together, making it challenging to understand their differences and unique applications. So, what exactly is an AI agent, and how does it differ from LLMs that seem to have boundless capabilities? Let’s break it down.
In short, an AI agent is a purpose-built system designed to perform a specific task or solve a defined problem. It achieves this by leveraging any or all the foundational building blocks of AI, including:
– Natural Language Processing (NLP): Understanding and generating human-like text.
– Optical Character Recognition (OCR): Converting scanned or printed text into machine-readable data.
– Large Language Models (LLMs): Providing language understanding and generation.
– Retrieval-Augmented Generation (RAG): Integrating external knowledge sources to ground outputs in real-world facts.
An AI agent is tailored to excel at one job. It is not a generalist but a specialist, designed with precision to deliver consistent, accurate, and actionable outputs for its specific domain.
LLMs, like OpenAI’s GPT, are vast, versatile, and capable of performing a wide array of tasks across different fields. However, their broad capabilities come with inherent challenges, such as:
- The need for precise and often progressive prompting to achieve desired outcomes.
- A propensity to generate plausible but incorrect information (commonly referred to as “hallucinations”).
An AI agent, on the other hand, is engineered with a narrower focus. It achieves its task-specific precision through a structured approach that includes:
- Seamless Input Integration:
– Ensuring that the input method aligns with the task.
– Examples include OCR for document scanning, NLP for language processing, or enterprise data integration.
- Prompt Engineering:
– Crafting specific and impeccable prompts tailored to the agent’s function.
– These prompts guide the AI’s responses, ensuring relevance and accuracy.
- Grounding in Enterprise Knowledge:
– Utilizing RAG to connect with an enterprise’s body of knowledge.
– Ensures outputs are contextually relevant and anchored in reality.
- Consistent Output Design:
– Standardizing the output format to be clear, visually appealing, and easy to interpret.
– Examples include reports, summaries, or dashboard data.
- Feedback Mechanism:
– Incorporating a feedback loop to enable fine-tuning and continuous optimization.
– Allowing the AI agent to learn from real-world performance and improve over time.
Practical Applications
AI agents are designed to excel at specific tasks, such as:
– Processing insurance claims.
– Automating and optimizing customer service.
– Extracting insights from a company’s proprietary data.
– Ensuring Regulatory compliance and conducting Gap analysis between laws and internal procedures.
While LLMs provide flexibility and broad utility, their lack of task-specific design can lead to inefficiencies in complex enterprise use cases. AI agents fill this gap by being fine-tuned and engineered to deliver high performance within a specific context.
AI agents often utilize LLMs as a foundational component but enhance their reliability and effectiveness by incorporating additional technologies like RAG and prompt engineering. By narrowing their focus, AI agents transform LLMs vast capabilities into finely tuned, task-oriented solutions. This synergy enables businesses to harness the best of both worlds—the power of LLMs and the precision of AI agents.
In conclusion, an AI agent is a finely tuned collection of tools incorporating many elements, including AI components, to excel at a specific task. By combining technologies like NLP, OCR, LLMs, RAG, and prompt engineering, and by focusing on seamless input integration, consistent output design, and continuous feedback, AI agents deliver highly specialized and optimized performance. In contrast, LLMs offer broad capabilities but require careful guidance to achieve specific results. Together, these technologies provide a comprehensive toolkit for solving diverse challenges in today’s AI-driven world.