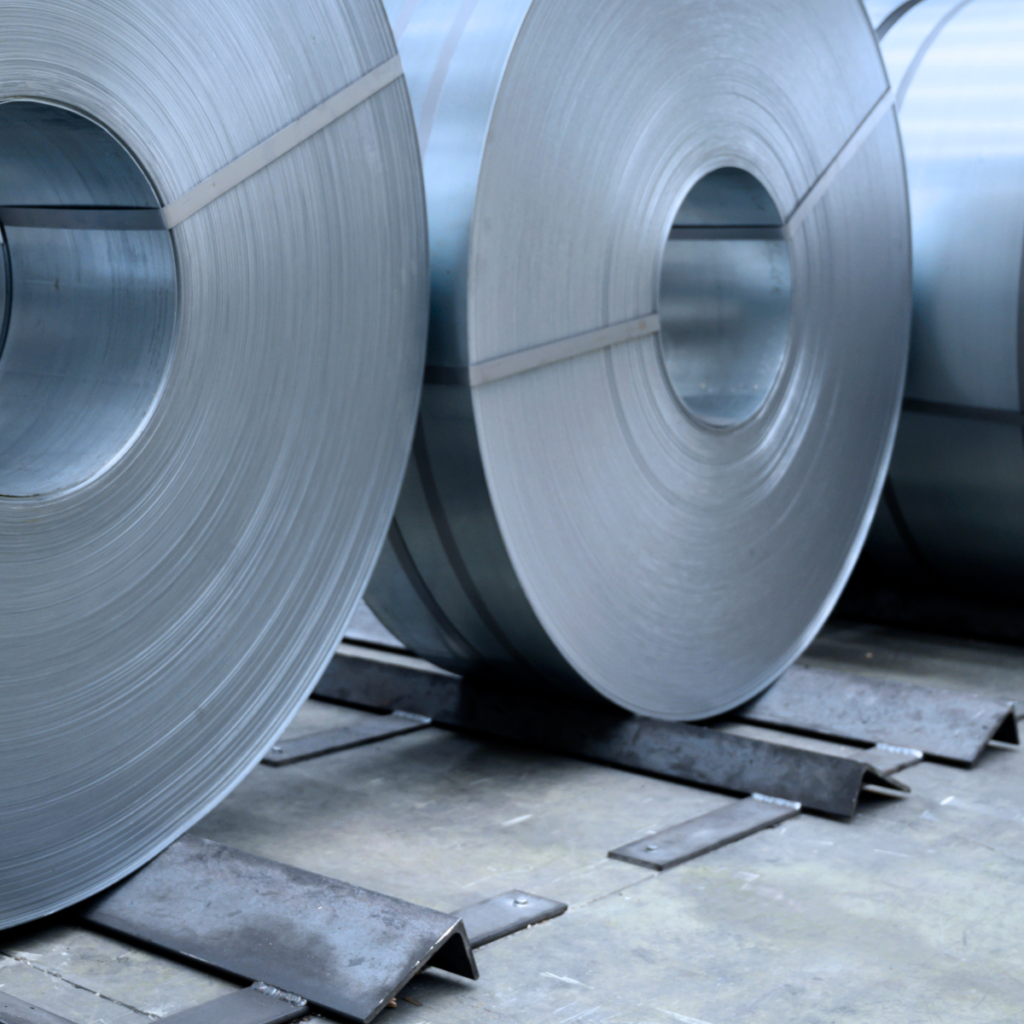
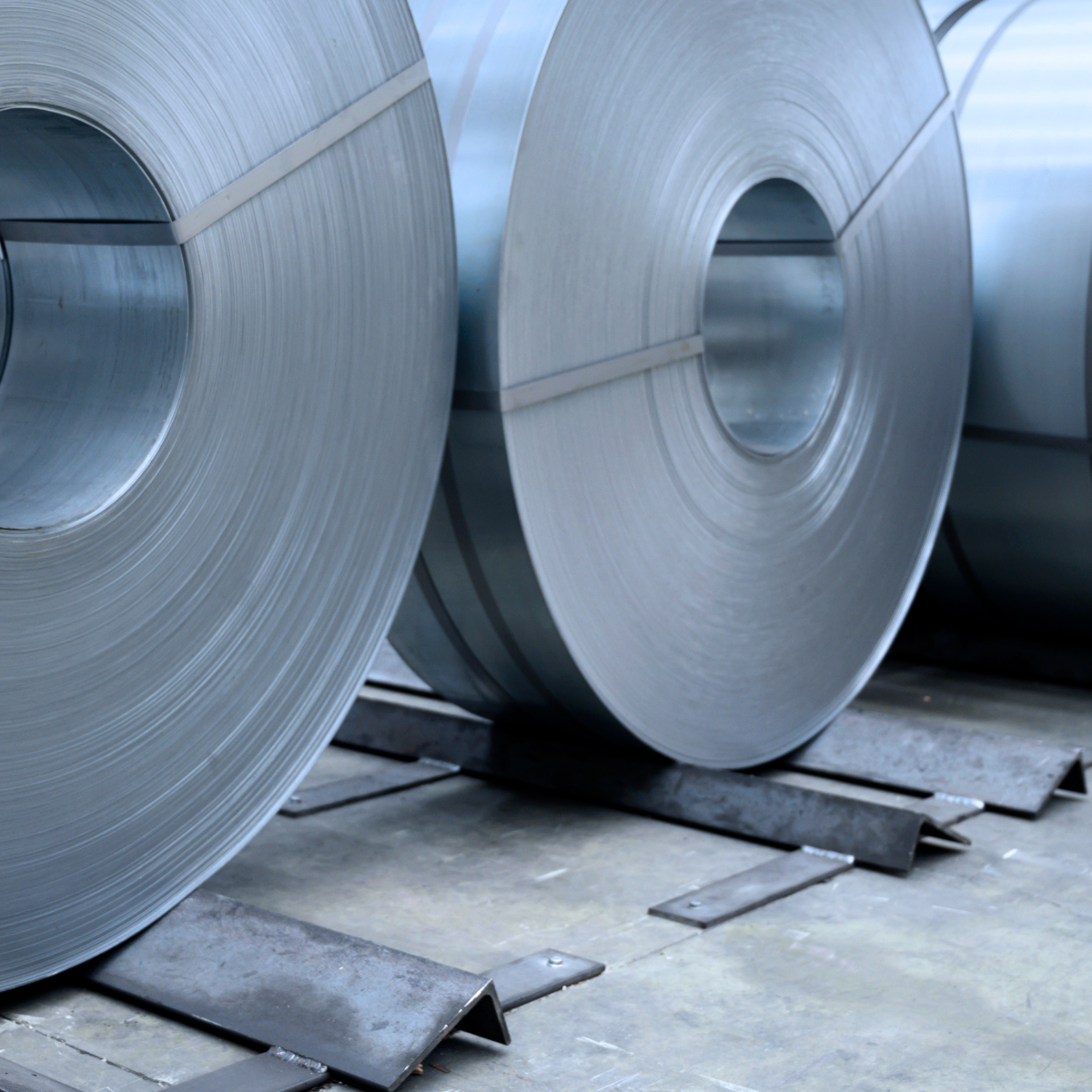
OVERVIEW
- A $75M USD alumina manufacturer was living with inconsistent production schedules due to constraints in measuring a key process
- The plant had to either wait for data or make preemptive adjustments that ended up over-correcting
- An ML model backed with engineering theory and deployed to the client’s servers with a user-friendly interface, put the measure at the operators’ fingertips leading to significant impact on the bottom line.
CHALLENGE
- The primary challenge was identifying all parameters that affected particle growth. While the process team was aware of the textbook factors, real world conditions added many more complications.
- Data was widely fragmented. There were data in network-gapped production machines, in laboratory databases, and several excel files where manual interventions were noted daily.
- The new interface had to be made highly user-friendly to keep the learning curve very short for operators and to ensure no interruption in production.
APPROACH
- Significant time was given to exploration of the production process. With our experience, we revealed over 30 additional plant actions and process parameters that directly affected growth.
- Data engineering worked with current structures and ensured minimum operational changes.
- Over 200 experiments and iterations resulted in an XGBoost machine learning model with 94% accuracy
- Our proprietary Explainability module reveals the reasoning behind the model’s predictions. This enables the plant to zero in on the root cause and take effective corrective and preventive actions